Senior Kathleen Zhang Named Scholar For Regeneron Science Talent Search
Kathleen Zhang, a current senior, was named as one of 300 scholars for her project in the Regeneron Science Talent Search.
Reading Time: 4 minutes
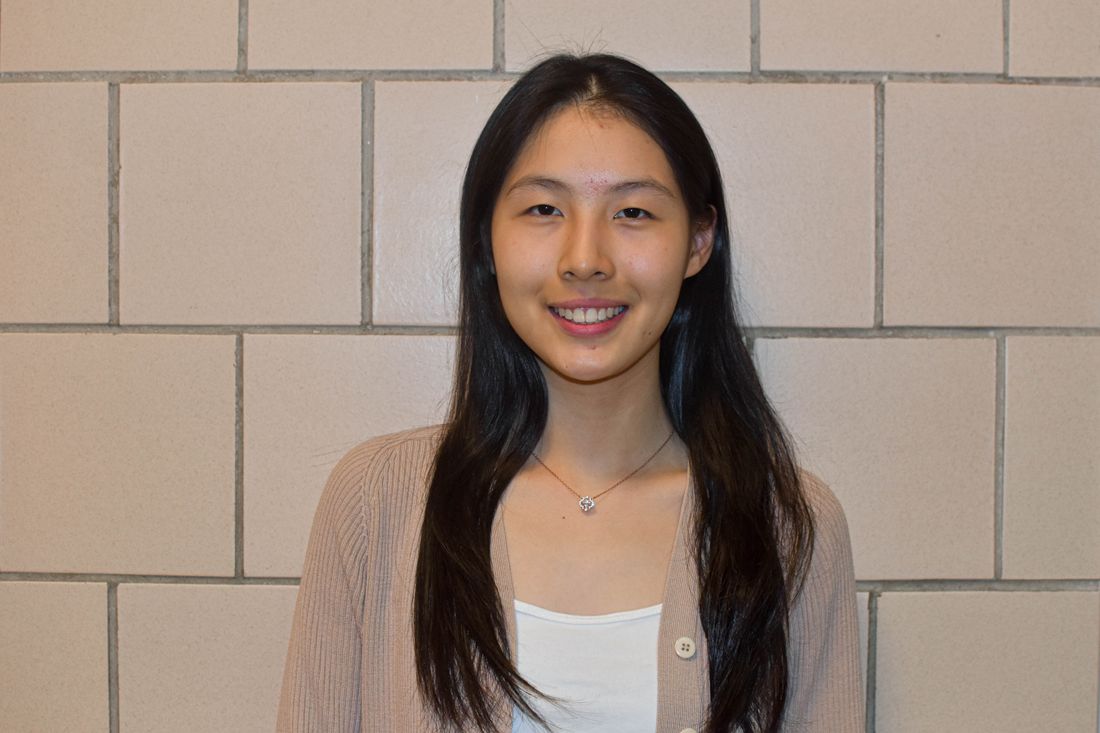
In collaboration with the Science Department
Additional reporting by Ziying Jian.
Senior Kathleen Zhang was selected as one of the 300 scholars in this year’s Regeneron Science Talent Search (STS) on January 10 for her project “Temporal Regression Method for Removing Hemodynamic Signal During Wide-Field Fluorescent Imaging of Epileptiform Activity.” Each year, STS invites student competitors to submit original research in important areas of scientific and mathematical study. Beginning in 1942, STS has encouraged students to develop ideas to solve societal problems, ranging from climate change to significant illnesses. At Stuyvesant, STS has become an opportunity for students to present their research interests and compete for awards of up to $250,000.
Zhang’s project focused on epilepsy and its effects on the brain through mathematical methods and data analysis. As with all scientific research, she experienced setbacks during her journey. Since she was unable to join the Regeneron class at Stuyvesant, she could not access the research opportunities it offered; instead, she had to seek out her own. “I think it’s very hard to start a project on your own with no background [and] no resources,” Zhang said. She reached out to professional scientists and mentors to guide her project, working closely with science teacher Jason Econome.
Zhang began her research journey by searching for laboratories online, where she soon came across a list of establishments specializing in her research area. Due to her interest in neuroscience, Zhang looked for laboratories that specialized in fluorescent data analysis of the brain. “The [Epilepsy lab at Cornell University Department of Neuroscience] really interested me because it [allowed me to] use my math background and apply it to this science topic. I’ve always been fascinated by the brain,” Zhang said.
Eventually, she found Dr. Hongtao Ma, who has been specializing in the mechanisms of epilepsy at Weill Cornell Medical College for almost 20 years. Zhang reached out to Dr. Ma because of his extensive research experience in mathematical models, computer programming, and optical pathway design. He helped Zhang by recommending specific mathematical projects for her to base her research on. “[Zhang] expressed her interest in my lab and sent her resume through e-mail. […] [Zhang] is a math expert and I assigned her a project to design a mathematical method for data analysis,” Dr. Ma said in an e-mail interview. “[She] discussed [her progress] with me routinely […] and [I] made sure she followed the right research direction.”
Zhang’s study required her to observe the brain undergoing epileptic activity. To observe this on a cellular level, scientists use wide-field fluorescent imaging to stain the chosen areas in individual cells with fluorescent molecules called fluorophores. When viewed through a filter that only admits certain wavelengths of light, specific areas of the brain are highlighted during an induced epileptic seizure for easy observation. However, the data obtained through fluorescent imaging experiments contain hemodynamic noise: changes in blood flow and oxygenation due to neurons becoming active in different regions of the brain. These signals can interfere with the accuracy of fluorescent imaging and cause changes in the absorption and scattering of light within cells, altering the amount of light that reaches and is subsequently emitted by the fluorophores. This can make distinguishing between true fluorescence signals and hemodynamic artifacts difficult. For instance, when hemoglobin absorbs light, its effects significantly impact fluorescence measurements over a broad spectrum of wavelengths.
Previously, a common method to filter the hemodynamic noise was based on Beer-Lambert’s Law. This law helps calculate how much light is absorbed by a sample and the intensity of the light emitted by fluorescence, which is directly proportional to the intensity of the incident light. However, the Beer-Lambert-based method does not remove all of the hemodynamic noise and thus leads to strange, paradoxical results.
In her effort to find a better method, Zhang uncovered the source of the problem with the Beer-Lambert method. The light signal carrying hemodynamic information was also carrying “DC noise”, otherwise known as direct current noise, which she discovered was the source of the strange results. Filtering the DC noise data further required a way to determine how much was present.
Zhang’s solution was to use a temporal regression, a type of statistical analysis that looks at how different variables change over time and relate to each other. It mathematically identifies relationships and trends among the variables. Experts use these models to make predictions and draw conclusions about similar data sets. Zhang built her model to plot fluorescence and hemodynamic data simultaneously. The y-intercept of this regression is the noise that should be subtracted from the data; this subtraction removes the hemodynamic noise entirely.
Zhang’s knowledge of mathematical models, coupled with her understanding of the data she collected, created a new method—one that will generate more accurate readings of cells and advance the research and treatment of neurological disorders.
Ultimately, Zhang is proud of her accomplishments. She feels that this experience was beneficial to her because it allowed her to explore her interests in a practical and applicable way, giving insight into fields she may want to explore in the future. “I got into an area of math and [computer science] that I wasn’t into before,” Zhang said. “This is my first time diving into applied mathematics [and] applying it to real world issues. I definitely enjoy this a lot, and this is probably the direction that I would want to go in the future.”