When AI Goes Wrong: The Healthcare Gamble
The increased reliance of AI, as even small errors or “hallucinations” by AI systems can ultimately harm those who depend on accurate medical care.
Reading Time: 3 minutes
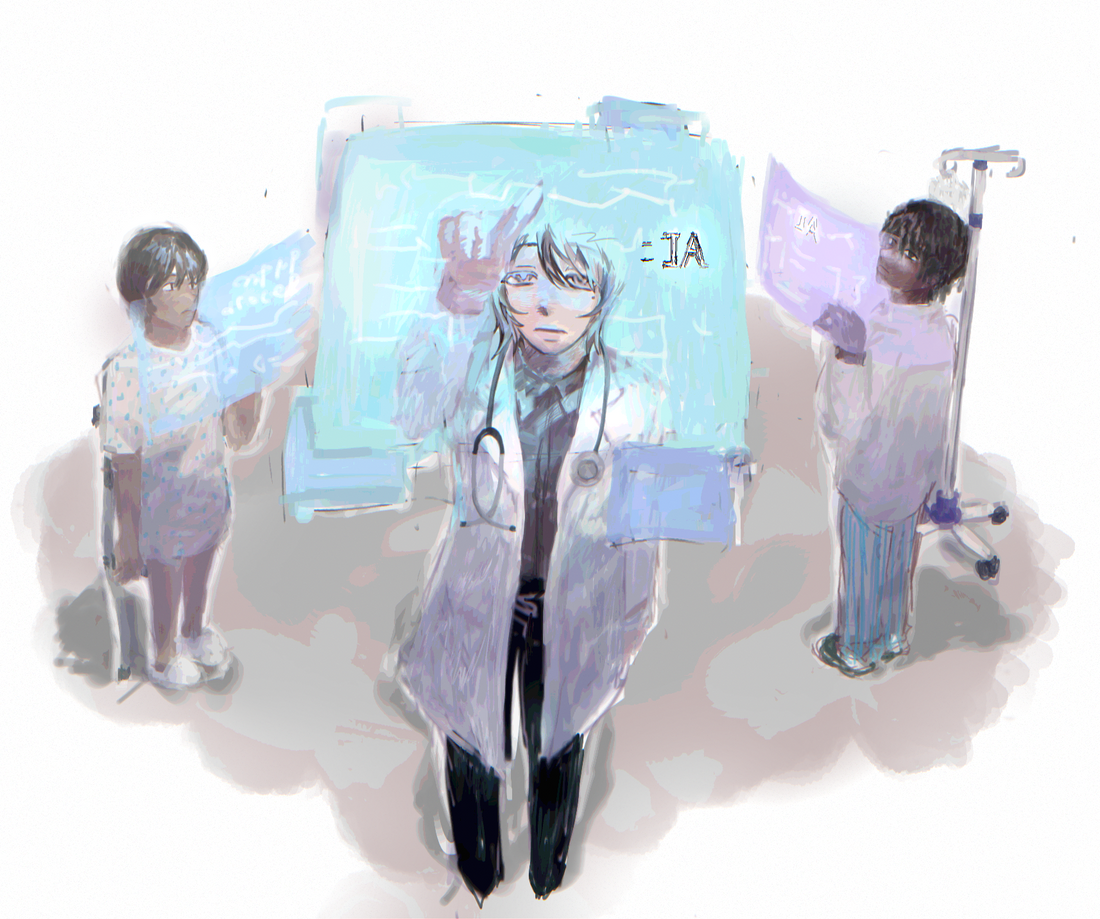
It’s the Sunday before school starts, and right before sending an email to your science teacher, you run your message through the AI grammar-correcting website, Grammarly, to make sure you don’t embarrass yourself with some silly grammar mistakes. Within three clicks of a button, your email is clear of typos, and it’s now perfect for sending. However, what if Grammarly were to give you a suggestion that appeared reasonable, only for it to be completely incorrect? In the context of sending an email, the stakes are low, but imagine the repercussions when Artificial Intelligence (AI)—generative models that can produce high quality content based on training data—is used in healthcare to diagnose patients whose lives are at risk. Thus, as we rely on AI as a tool for our everyday lives, it’s crucial to acknowledge that while AI’s potential in medicine is remarkable, it has serious implications for patient safety. Even small errors or “hallucinations” by AI systems can lead to misdiagnoses, improper treatments, and ultimately harm to those who depend on accurate medical care.
AI hallucinations are false content generated by AI that is seemingly presented as factual and can appear in many different areas of the healthcare industry. They can create diagnostic errors, inaccurate patient records, and incorrect medication dosages. By fabricating evidence to back up scientists’ hypotheses, such as creating flawed connections between two unrelated subjects, there is a clear lack of explainability—the lack of transparency behind the functionality of a model—that comes with AI. Especially in a biomedical setting, explainability acts as an important device as it can help interpret the logic behind the content that AI has produced to ensure it can be utilized to quickly recognize flaws in the typical patterns that AI creates. Therefore, when there’s a lack of explainability, it becomes difficult to connect the logic between two ideas and mistrust increases between the fields of technology and medicine.
In addition, insufficient or biased data can be harmful to patients of minority demographics. This is not only prevalent in the usage of generative AI in medicine—there has been a recurring issue of AI perpetuating skewed biases based on gender and race. For instance, in a Gender Shades project conducted by Buolamwimi in 2017, AI was tested among commercial gender classification systems. It was concluded that while these systems performed better on male, white skin, there were significant error rates between that of darker skinned females, with a notable 50 percent higher error rate. This indicates that many AI models fail to represent minority groups and that AI’s current systems risk reinforcing existing social biases which can potentially cause harm to marginalized communities in healthcare.
While these hallucinations produce multifaceted effects, these technical errors arise due to fundamental limitations in AI training methods, such as insufficient datasets, complex models, and systemic gaps in representing the human majority. For instance, generative AI models are trained from an immense source of internet data that holds the information that they are trained to mimic, including any false information that is integrated into it. In the context of medicine, studies have shown that in the preoperative phase of anesthesia, AI is heavily relied on to monitor communication between teams and to predict the outcome of the operation. Therefore, the occurrence of AI hallucinations can have a substantial impact as it can directly impact surgical strategies where the patients lives are at risk. In addition, AI models are limited by their training to predict the next concept without the ability to verify the accuracy of their content, which contributes to why hallucinations are produced. In fact, in a University of Michigan study, Associate Professor of Computer Science Jenna Weins evaluated the accuracy of AI in medical diagnosis, finding that when given real clinical X-rays of patients with respiratory failure accompanied by AI explanations, clinicians were still not always able to detect when AI provides biased advice. The study found that when AI models were intentionally biased, especially when using irrelevant features like age or bone density to predict conditions like pneumonia, clinicians still relied on the model’s flawed reasoning, leading to incorrect diagnoses. This demonstrates that the imperfections that come with AI-based training can later impact its responses, giving AI a less reputable position in medicine.
With AI reliance starting to increase in healthcare, it becomes increasingly important to acknowledge the risks associated with using such algorithms. Thus, healthcare professionals have taken recent steps to mitigate misinformation by AI. For instance, many universities and hospitals have expanded their CME courses to include the issues of AI in healthcare. In addition, responses created by AI are always cross-checked in clinical settings with peer reviewed resources. These are among the many steps to try to control what is known to be such a transformative technology into something that can be used safely.