Unlocking the Secrets of GPT3: An In-Depth Look at How It Works
OpenAI logo with a bright and shining light behind it
Reading Time: 4 minutes
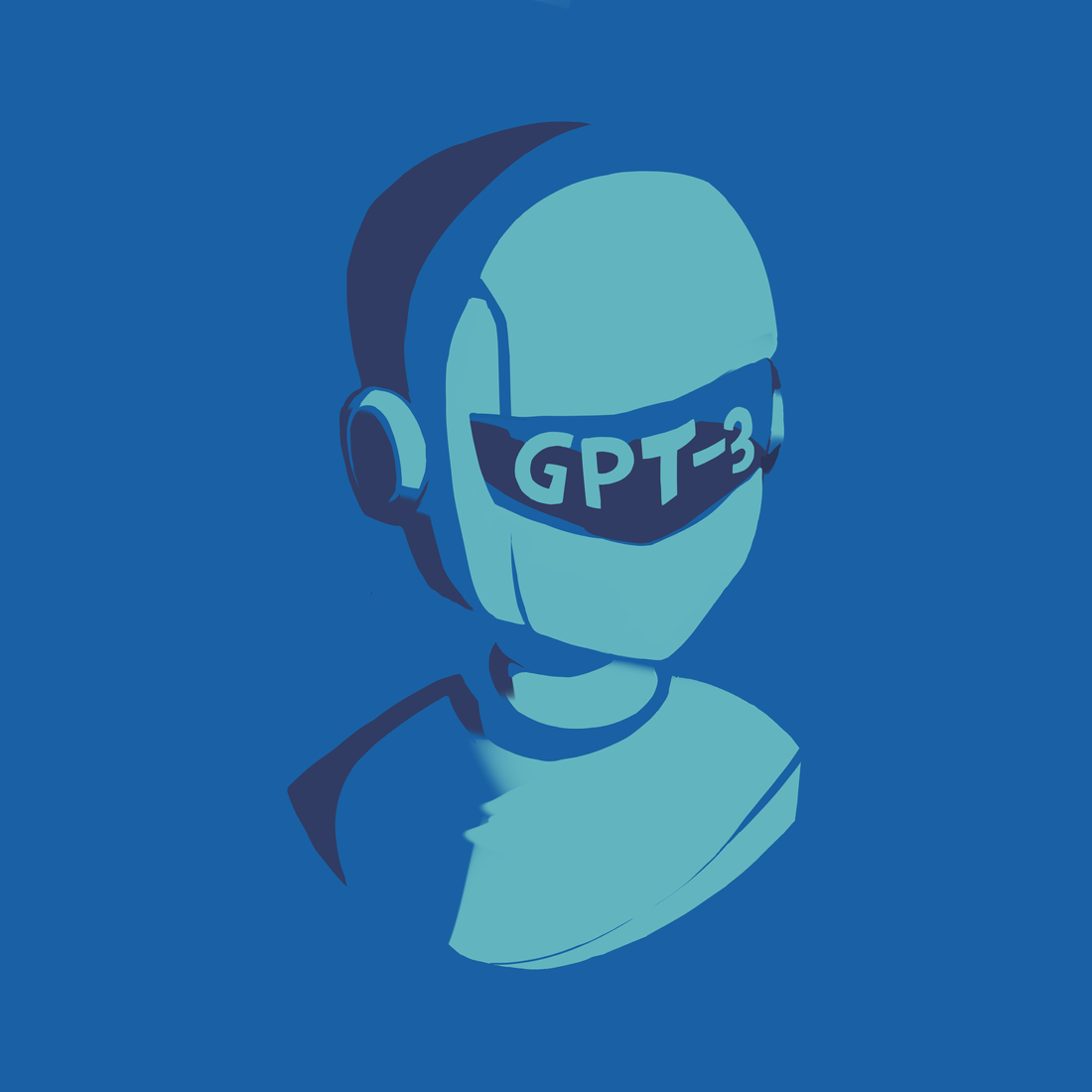
Have you ever wanted a supercomputer that could code, give medical advice, and help you study for tests? We have one in our hands, and its name is GPT-3. Its creator, OpenAI, a company co-founded by Elon Musk in 2015, has been making headlines for advancing artificial intelligence. Gaining worldwide fame in 2020, many of OpenAI’s artificial intelligence (AI) innovations, like DALL·E 2, have skills that millions are certain will change the world. Yet, the most famous and advanced OpenAI invention is GPT-3. Its sister app, ChatGPT, has gained one million users in a record-breaking five days. However, one must ask themself: how exactly does this AI work?
GPT-3, or Generative Pre-trained Transformer 3, is the world's largest neural network with 175 billion parameters. The parameters are mostly from Common Crawl, a non-profit organization that crawls the web and puts information into a database. GPT-3 is mainly used to generate text for summarization and question answering, with some calling it a “Google killer.” But as the name implies, GPT-3 is not the first AI released by OpenAI. In fact, to find out how GPT-3 works, we must first take a look at its predecessors.
Before GPT existed, there was Natural Language Processing (NLP). The goal of NLP was to allow people to use common languages to converse with a machine, which was further advanced by GPT-1. Released in 2018, the first model was trained on eight million websites and had 1.5 billion parameters. It used a “transformer model,” which made some words matter more than others and allowed people to give it random text. For example, if a user was to ask the program for a weight loss plan and provided their weight and favorite color, the program would prioritize the information about their weight. GPT-1 also used “unsupervised learning,” a type of training in which the AI has to find patterns between words using given data. The release of GPT-2 in 2019 took it a step further. With 15 billion parameters, it used "dynamic control," which allowed the AI to decide which information given was important to its response. If a user asked for instructions on how to make a necklace and then mentioned that they were wearing a blue shirt, it would know that such information is irrelevant to the user’s request for instructions.
The latest model, GPT-3, was released in 2020 and changed the AI scene completely. Aside from being the largest AI model released, it uses a creation of OpenAI called “dynamic batching.” That allows the AI to split up words into different batches for efficient processing, as word input varies.
Though GPT-3 is remarkably advanced, ChatGPT, OpenAI’s newest AI, surpasses it. ChatGPT is smaller than GPT-3 and uses a new technique called Reinforcement Learning from Human Feedback (RLHF). RLHF is an efficient form of unsupervised learning. When it is training, it receives a digital reward or punishment, which helps quicken the training process significantly. With 175 billion parameters and the large size of GPT-3, imagine how powerful ChatGPT could be.
Despite their advanced technology, GPT-3 and ChatGPT have their restrictions. For one, OpenAI has prevented both AIs from advising on how to make weapons. However, it can be bypassed quite easily, as many people were easily able to do so by tricking the AIs. They would tell ChatGPT to write a script between someone teaching a dog how to make a gun and thus bypass the limit. While the AIs seem to be extremely intelligent, ChatGPT fares poorly on the SAT. It scored 1020 out of 1600, failing to beat the national average of 1060 and Stuyvesant’s average score of 1510. Lastly, OpenAI’s AIs are unable to provide good information on niche topics. For example, when I asked ChatGPT to give me a summary of an ancient Chinese warlord named Cao Cao, it outputted many errors.
Despite being the largest neural network in the world, GPT-3 has the potential to be even bigger. With rumors of GPT-4 being released within the next year, it will certainly be interesting to see what new capabilities it will have. GPT-4 is rumored to release 500 trillion parameters, which is more than GPT-3’s 175 billion. This would allow GPT-4 to generate even better text, and do more tasks that require specific knowledge, like giving medical or law advice.
While we wait for GPT-4, there are things that we as users can do to enhance GPT-3, such as fine-tuning GPT-3 for specific tasks. Fine-tuning is when you add more information to GPT-3, specifically about a certain topic or area. This allows the AI to be an expert in a particular area, while still having general knowledge about everything else. For example, you could add a large amount of historical information to GPT-3 and train it to respond like a historian. Or you could add a large amount of information on food to GPT-3 and use it to find new healthy recipes.
However, if fine-tuning is too complex for you, there is another way to make GPT-3 respond the way you want. A process known as “prompt engineering” allows you to specify how you want the AI to respond. Instead of adding information to GPT-3, you give GPT-3 instructions on how you want it to respond. If you want GPT-3 to respond to you like it is in an anime rom-com, tell it to act like a character in an anime rom-com. Alternatively, if you want GPT-3 to respond like a teacher grading your essay, you can tell it to act like a harsh English teacher that gives great feedback.
GPT-3 is the most advanced neural network, and OpenAI has advanced the AI industry tenfold with new innovative ways to train its AIs. It is already changing the world, with NYC schools banning ChatGPT on school wifi. However, OpenAI can always create better AIs, either by making them bigger or by making them learn more efficiently. However, with how AI has been advancing, it would not be surprising if one day, GPT becomes the backbone of trillion-dollar companies.