How A.I. is Shaping the Future of Agricult
As a scientific research field that gained vast popularity in recent years, artificial intelligence (A.I.) has begun to expand its footprint into one of the oldest foundations of humanity—agriculture.
Reading Time: 3 minutes
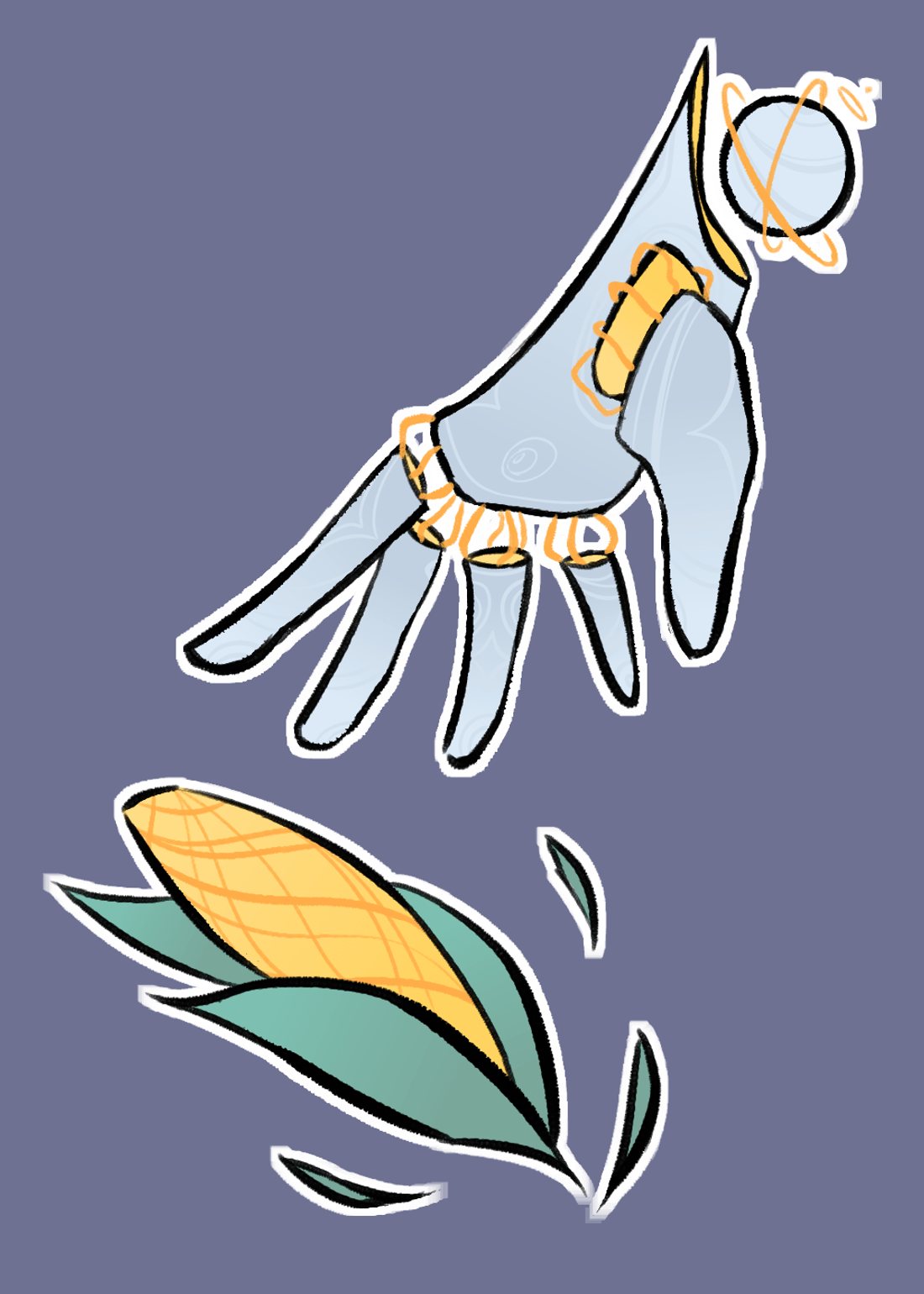
When you stop by the grocery store to grab a bag of freshly grown tomatoes for dinner, your eyes will likely be drawn to the tomatoes’ country of origin and expiration date on the front cover of the package. There is, however, another aspect of the production of these tomatoes that only a few individuals will pay attention to—these tomatoes may have once been under the hands of nonhuman entities: artificial intelligence (A.I.).
As a scientific field that has gained vast popularity in recent years, A.I. has expanded into one of the oldest foundations of humanity—agriculture. With the world’s rapidly increasing population, farmers worldwide are under immense pressure to increase their crop yield and agricultural biodiversity to meet growing consumer demands. Coupled with economic shutdowns due to COVID-19, the global food system must be transformed to provide affordable, healthy diets that are sustainable and inclusive in efforts to eradicate hunger, food insecurity, and malnutrition. Thanks to the emerging developments in A.I. technology and machine learning, the productivity of farms has increased significantly, along with the minimization of resource and land usage.
One of the most common applications of A.I. in agriculture is the creation of autonomous robots to monitor and manage essential agricultural activities and environmental factors much faster than that of the traditional, manual approach. NatureSweet, for example, is a San Antonio-based grower of premium fresh tomatoes that uses machine learning and computer vision, a field of A.I. that trains computers to interpret the visual world, to increase crop yields and control pests and diseases in its greenhouses. To do this, the company implemented task-tracking systems to monitor watering and seeding. Ten cameras are installed in the greenhouse ceilings to take photos of the crop and provide instant feedback. By detecting insect infestations or other problems, weekly harvests are estimated to grow by two to four percent, and the company will produce healthier tomatoes every day.
In addition to ground data, seasonal forecasting and predictive analytic models are commonly used to improve agricultural accuracy and productivity. Under changing climate conditions and increasing pollution, it is often quite difficult for farmers to determine the right time to sow seeds, water, and harvest. A.I.-enabled technologies, however, can assist farmers through accurate predictions in upcoming weather patterns and environmental conditions. Both seasonal forecasting and predictive analytic models have become crucial tools used in small farms, especially in developing countries. As A.I. continues to spread, these small farms grow beautiful yields and create a lasting impact on the global food supply chain, accounting for approximately 70 percent of the world’s crop production.
Coupled with unmanned aircraft systems and drones, A.I. can capture images and data points of the entire farm, allowing farmers to analyze a wide spectrum of environmental factors—including temperature, water usage, and soil conditions—in near real-time. More importantly, these captured images will then be analyzed through deep learning algorithms and neural networks where farmers can quickly identify problem areas, such as those being affected by pests or bacteria, and receive potential improvement suggestions generated by the A.I. model. This particular aspect of A.I. in agriculture is especially valuable in farms that stretch across large areas since unmanned drones can cover far more land—sometimes thousands of acres—in a much shorter time than humans on foot.
Additionally, a general management style called precision agriculture often uses A.I. sensors to collect and analyze data. For instance, it can target diseases and pests in crops before the application of herbicides to prevent overuse and pesticide resistance. Farmers also use precision agriculture to implement probabilistic models for determining the best crop varieties for future growing seasons and their most ideal planting times and locations.
Crop diseases account for the primary causes of deprivation and food security concerns. The International Center for Tropical Agriculture (CIAT) is an international research and development organization that aims to improve the livelihoods of farms and reduce poverty and hunger in developing countries. In the CIAT agricultural biodiversity research division, scientists have used A.I. technology to create a smartphone program to help detect the signs of five major diseases and one common pest found in banana plants. With an average 90 percent successful detection rate, this platform is a remarkably useful built-in tool in a mobile application called Tumaini, which helps smallholder banana growers monitor their crops and prevent a large-scale banana disease outbreak. There, the app imports a large dataset of banana disease images taken in Congo, Uganda, and South India to generate a series of recommendations and management techniques.
Beyond the current applications of A.I., there are many other unique technological advances making notable headway in agriculture. A.I.-controlled vertical farms, for example, require significantly less land and water resources compared to traditional farming operations due to the use of robots to monitor growth patterns and variables of water, temperature, and light. With that being said, it is unquestionable that the future of agriculture will intertwine with developments in A.I., but the outcome of this crossover will remain a mystery for decades to come.