Can You See Me Now?
Facial recognition and the data sets it uses must be regulated by the government so that racial, gender, and class biases do not persist, and so that minorities are not unfairly persecuted.
Reading Time: 3 minutes
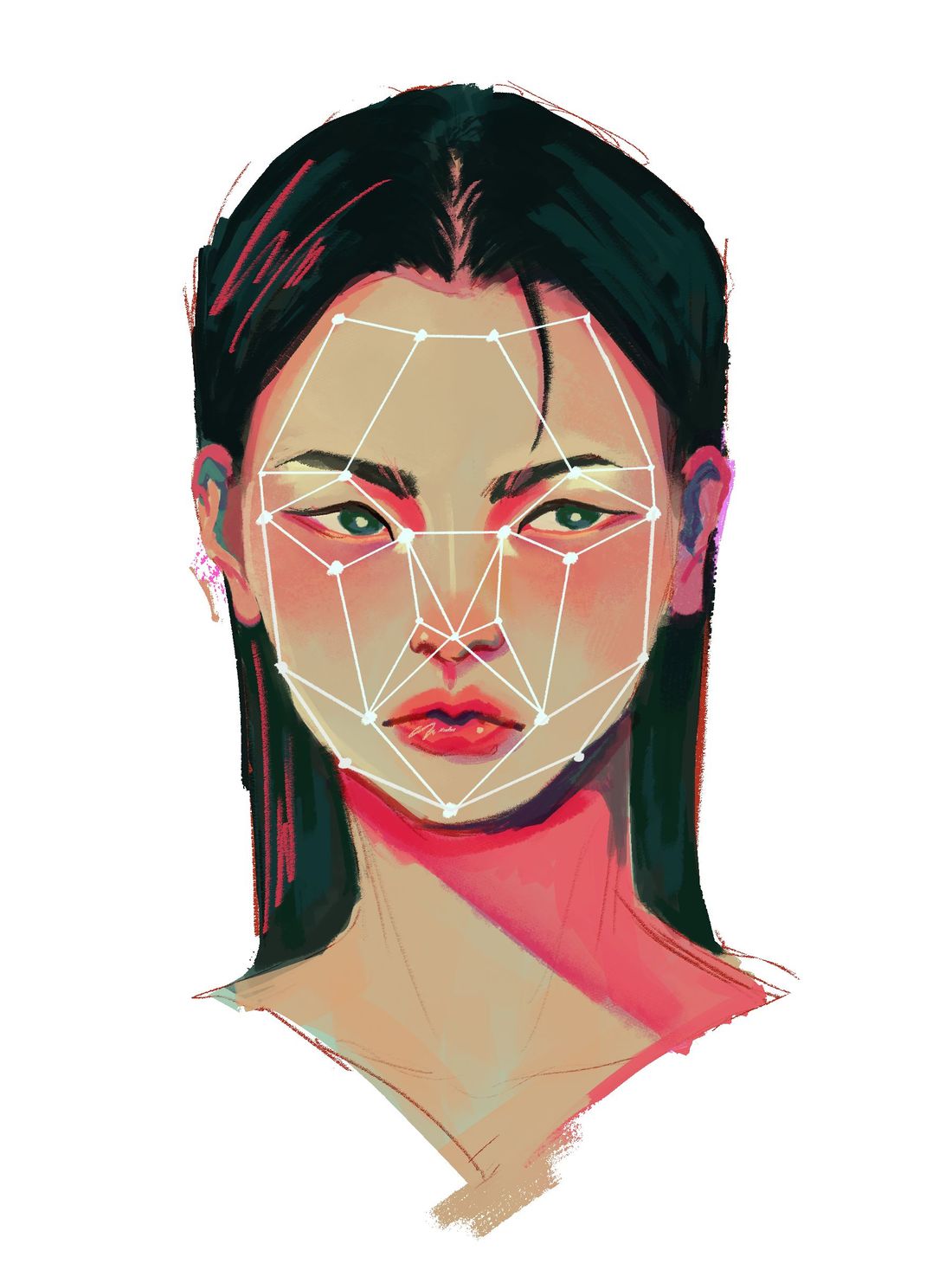
There are many methods by which companies use technology to ensure who you are: passcodes, fingerprints, security questions, and voice recognition are some of the most common examples. Among these, facial recognition is one of the leading technologies for security and identification. It relies on algorithms and large databases of human images to create patterns that are used to identify unique features. The methods computers use are “black boxes,” meaning that programmers cannot predict how computers identify certain patterns, but that mechanism doesn’t mean that they are unbiased.
The earliest form of facial recognition was developed in the 1960s by Woody Bledsoe, Helen Chan Wolf, and Charles Bisson. The program involved marking certain landmarks that most human faces should have, such as eyes, mouths, and noses. However, it is hard to express these uniquely varying body parts using binary ones and zeroes, meaning that we cannot just tell a computer, “If you see (blank), that is a nose. Now color it.” In order for the computer to recognize these features, programmers use a different strategy: machine learning, which involves giving a computer a set of data—in this case, it is a bunch of photos of your normal, average Joe—in the hopes that the computer will pick up on the patterns you want them to. Programmers will not always know how and why a computer picks up on the patterns it does. The computer might notice things that are overlooked by human eyes, or it may never notice a pattern that we do. But despite the seeming lack of control, there are ways to trick the computer into ignoring certain patterns by eliminating entire sets of data completely.
By controlling the data sets that computers receive, programmers can, perhaps unconsciously, imbue their biases into facial recognition. This influence was uncovered in a 2018 study conducted by MIT and Stanford University. After testing three different facial recognition algorithms, programmers Joy Buolamwini and Timnit Gebru found that the most popular facial recognition algorithms recognized light-skinned males more accurately than dark-skinned males, and were worst at recognizing dark-skinned females. Deeper investigation found that the data sets given to these algorithms, such as Amazon’s, were incredibly skewed. Most pictures presented were of white men, and few pictures were of women of color.
In an era of technological advancement, this algorithmic bias is incredibly problematic, as facial recognition is being implemented in more than just phone lock screens. In the UK, government security organizations are placing cameras on the streets to identify criminals. People of color can be inaccurately identified as criminals and falsely accused of breaking the law. In China, facial recognition is used everywhere in public to identify those who protest the government and to identify Uyghur Muslims. While facial recognition is sometimes helpful for the police, it is a pervasive use of surveillance that is often used to target certain demographics.
Coded bias is a problem beyond just facial recognition. In the US, facial recognition and other machine learning algorithms are used to test applicants for mortgages, loans, jobs, and food stamps. Microsoft created an AI called Tay, a chatbot that learned from what it saw online, in 2016. Tay was coded to learn from its online interactions with social media users. Within 16 hours, Tay learned an entire slew of racist, mysoginistic, and xenophobic language that it then posted on Twitter. Because Tay and facial recognition are both machine learning programs, they use similar code and data sets. If we do not take the initiative to diversify facial recognition and prevent organizations from using them on unknowing consumers, countless minorities will be hurt financially and socially simply because they were not accurately represented in the datasets of a white male-dominated industry.
Action must be taken. Local governments must regulate the use of machine learning algorithms and forms of commercial facial recognition. One solution is to set up standardized data sets for facial recognition that companies must either use or mimic closely. These standards must represent people of different races, genders, and ages so that facial recognition algorithms know how to deal with different kinds of faces. However, we cannot ignore that biases will always be present because that skewing of data is simply the nature of all machine learning algorithms.
Most people have a hard time understanding that a computer can be biased, because we have constructed a narrative that machines are more reliable and safer than humans. In many cases, that comparison holds true. Facial recognition has made security more reliable and legal processes much faster. But as long as facial recognition is unchecked by the federal government, problematic biases will persist.